In the field of digital communication, steganography is a technique that embeds secret information into innocuous carriers to achieve secure communication—akin to the "Morse code" of the intelligence world. It aims to disguise secret information as ordinary data and transmit it through public channels. In recent years, generative models (e.g., GPT, DeepSeek, Stable Diffusion) have demonstrated groundbreaking capabilities in synthesizing high-fidelity data, opening new opportunities for steganography and giving rise to generative steganography.
The core challenge of generative steganography lies in making secret information a natural byproduct of the generation process rather than an artificially appended "foreign object." Among existing approaches, prefix-based steganographic techniques (e.g., Arithmetic Coding [AC], Meteor) have garnered significant attention due to their provable security. These methods leverage the probability distribution of generative models to encode secret information as a natural outcome of the generation path. However, existing solutions still face a classic trilemma: how to achieve an optimal trade-off among security (undetectability), capacity (embedding rate), and efficiency (computational cost).
To address these bottlenecks, Pan Chao (Ph.D. candidate; advisor: Prof. Hu Donghui) proposed a Security-Enhanced (SE) and Efficiency-Enhanced (EE) scheme. Their research, titled "Rethinking Prefix-based Steganography for Enhanced Security and Efficiency," has been accepted by IEEE TIFS 2025, a top-tier journal in information security and forensics. The corresponding authors are Prof. Hu Donghui and Associate Prof. Wang Yaofei.
Paper Title: Rethinking Prefix-Based Steganography for Enhanced Security and Efficiency
Authors: Chao Pan, Donghui Hu, Yaofei Wang, Kejiang Chen, Yinyin Peng, Xianjin Rong, Chen Gu, and Meng Li (Associate Prof. Chen Kejiang is affiliated with the University of Science and Technology of China)
Paper Link: https://ieeexplore.ieee.org/document/10919130
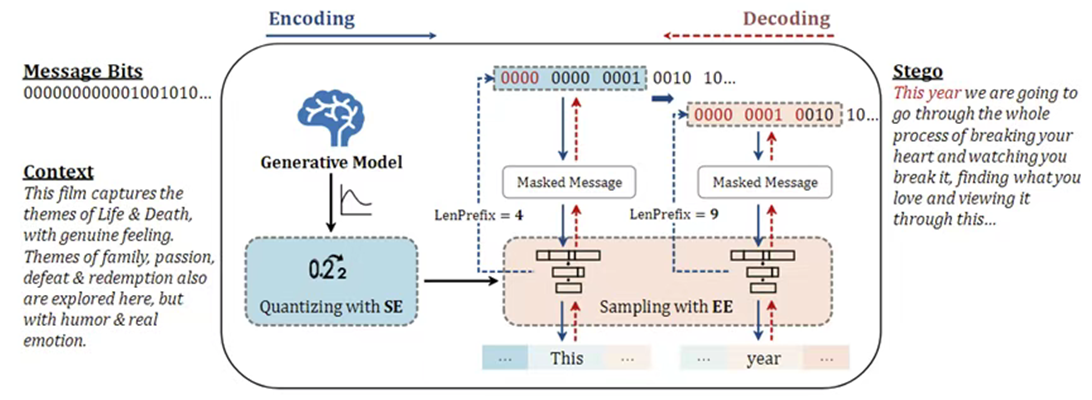
Fig. 1. Overview of prefix-based steganography with SE and EE. SE and EE are the two components we proposed to enhance the security and efficiency of prefix-based steganography, respectively
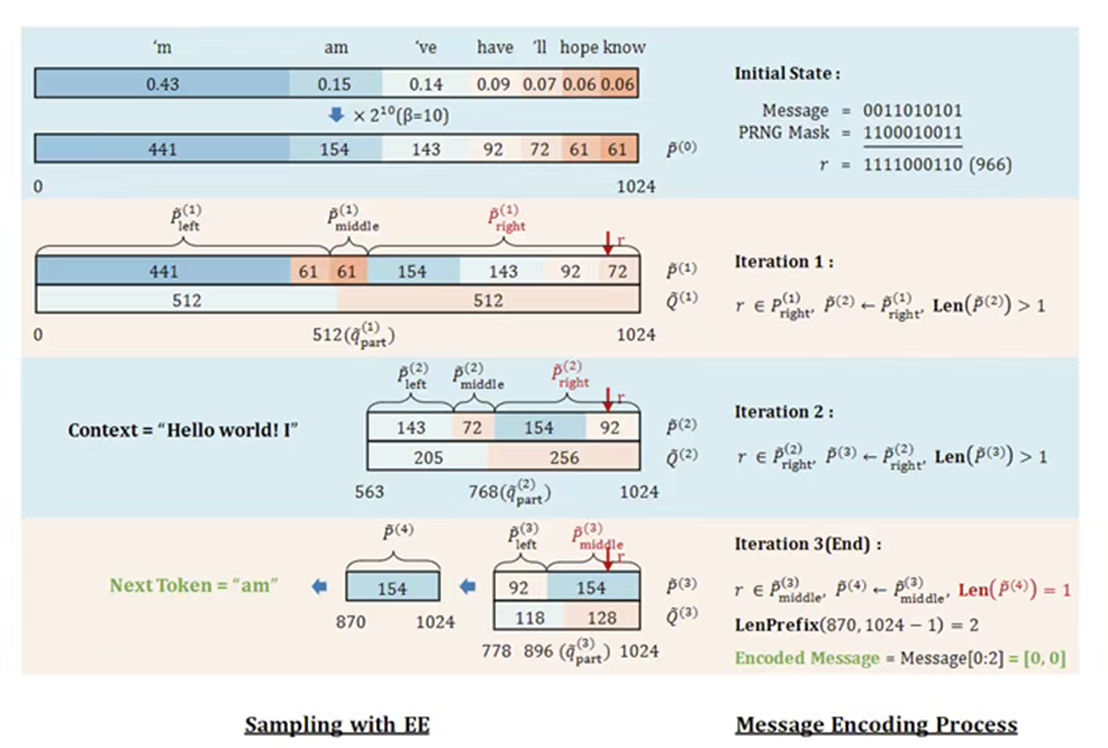
Fig. 2. An example of the sampling process of prefix-based steganography with EE component
Abstract: Generative models have demonstrated remarkable capabilities in synthesizing realistic content, creating new opportu nities for secure communication through steganography—the practice of embedding covert messages within seemingly innocuous data. While prefix-based steganography, which encodes secret messages into shared probability intervals during generative sampling, has emerged as a promising paradigm for provably secure communication, its practical adoption remains constrained by inherent tradeoffs between security, capacity, and efficiency. To address these challenges, we propose two enhancements. The first enhancement optimizes quantization distortion in existing frameworks to minimize KL divergence, thereby enhancing theoretical security. The second redesigns the sampling mechanism via distribution coupling to amplify steganographic capacity, achieving this without incurring substantial computational overhead. Experimental validation on text generation task confirms our enhancements substantially outperform previous implementations, demonstrating notable capacity improvements, marked security enhancements, and efficiency gains on consumer-grade hardware. Cross-task comparisons with popular provably secure steganography further establish the proposed enhancements as achieving superior security-capacity-efficiency tradeoffs across diverse generative scenarios, advancing the practical deployment of provably secure steganography systems.
IEEE Transactions on Information Forensics and Security (abbreviated as IEEE TIFS) is a premier academic journal in the fields of information security and digital forensics. Established in 2006 by the IEEE Signal Processing Society, the journal focuses on cutting-edge research areas including data security, applied cryptography, biometric recognition, and related advanced topics. Recognized as a Category A international journal by the China Computer Federation (CCF), IEEE TIFS maintains a 2024 impact factor of 6.3 and is classified as a Q1 journal in Computer Science according to the Chinese Academy of Sciences ranking system.