In today's digital era, data flows through every facet of our lives like an ever-present stream: from interactions on social media to medical records in healthcare systems, from financial transactions to sensory data in smart cities. Beneath this seemingly disorganized data lies a complex web of causal relationships, which are essential for understanding the world. These relationships shed light on how marketing strategies shape consumer behavior, how climate change impacts crop growth, and the intricate links between medical treatments and patient recovery.
Yet, extracting these causal relationships from vast datasets remains a formidable challenge for researchers. Traditional causal discovery methods typically rely on centralized data analysis, which often raises significant privacy concerns. As awareness of data privacy grows, the need to uncover causal relationships while safeguarding individual privacy has become a pressing issue.
Federated learning has emerged as a promising approach to addressing privacy concerns, but it still faces significant obstacles in practice. Variations in data quality across sources can compromise learning accuracy, while the computational complexity of federated methods often results in high resource costs.
To tackle these challenges, Professor Kui Yu and his doctoral student, Xianjie Guo, from our institute have developed two innovative frameworks for federated causal discovery. Their groundbreaking research has been featured at the top-tier artificial intelligence conferences AAAI 2024 and IJCAI 2024, offering new solutions to the longstanding challenges of causal discovery in federated learning environments.
Paper Title 1: FedCSL: A Scalable and Accurate Approach to Federated Causal Structure Learning
Authors: Xianjie Guo, Kui Yu, Lin Liu, and Jiuyong Li
Paper Link: https://ojs.aaai.org/index.php/AAAI/article/view/29113
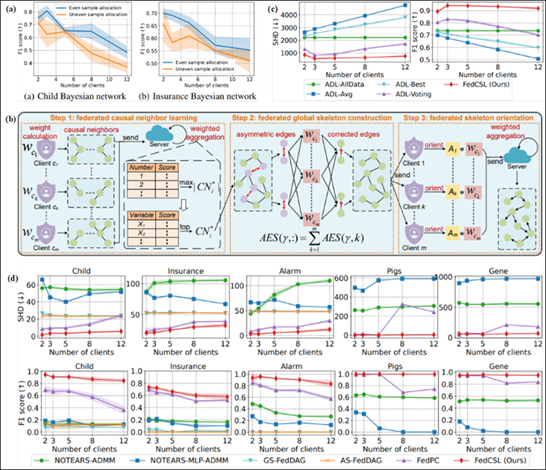
Figure 1 Explanation: (a) This subfigure illustrates the impact of sample distribution on performance. Keeping the total sample size constant, samples are distributed across clients in either a uniform or non-uniform manner. Under the same client weight assignment, existing methods perform significantly worse with non-uniform sample distribution compared to uniform distribution. (b) The proposed FedCSL framework, which consists of three main steps, is depicted here. (c) Experimental results of federated causal structure learning on a high-dimensional synthetic dataset are presented, showcasing the performance of FedCSL. (d) Experimental results of federated causal structure learning on a benchmark Bayesian network dataset are provided, demonstrating the effectiveness of the proposed method.
As an emerging research area, Federated Causal Structure Learning (FedCSL) aims to uncover causal relationships from distributed data across multiple clients while safeguarding data privacy. However, existing FedCSL algorithms face challenges in scalability and accuracy. These methods typically require computationally expensive causal structure learning algorithms to be executed on each client, which limits their scalability. Moreover, in real-world scenarios, the sample sizes across clients often vary significantly. Current methods assign equal weights to the structural information learned from each client, which severely impacts learning accuracy. To address these limitations, this paper introduces two novel strategies: 1. Federated Local-Global Learning Strategy: This strategy enables scalability to high-dimensional data. 2. Weighted Aggregation Strategy: It improves accuracy by incorporating varying contributions from clients without relying on complex cryptographic techniques, while still preserving data privacy. Extensive experiments on benchmark datasets, high-dimensional synthetic datasets, and real-world datasets validate the effectiveness of these two strategies.
Paper Title 2: Sample Quality Heterogeneity-aware Federated Causal Discovery through Adaptive Variable Space Selection
Authors: Xianjie Guo, Kui Yu, Hao Wang, Lizhen Cui, Han Yu, and Xiaoxiao Li
Paper Link: https://www.ijcai.org/proceedings/2024/0450.pdf
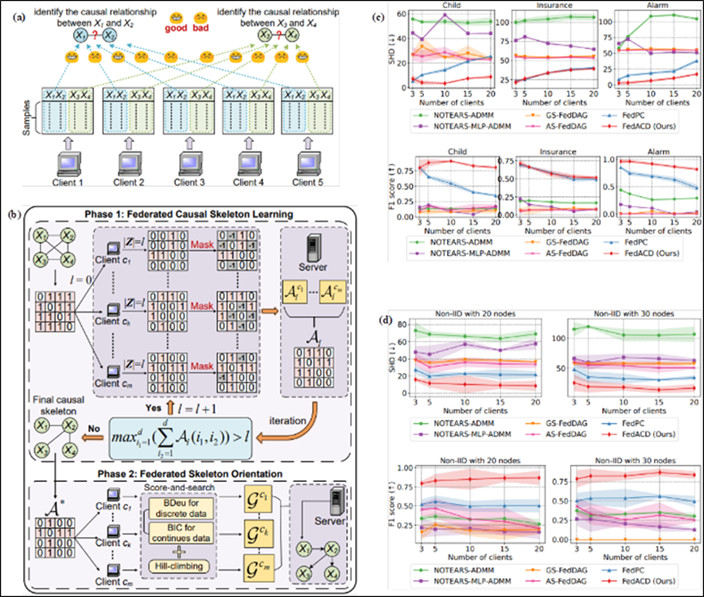
Figure 2 Explanation: (a) An example of sample quality heterogeneity in federated learning scenarios: For identifying the causal relationship between X1 and X2, only the samples from Clients 1 and 3 exhibit "good" quality within the variable space of X1 and X2 (i.e., they can correctly infer the causal relationship). Conversely, the sample quality from other clients in the same variable space is "poor" (unable to correctly learn the causal relationship). Similarly, for determining the causal relationship between X3 and X4, only the samples from Clients 2 and 5 demonstrate "good" quality within the variable space of X3 and X4, while the sample quality from other clients in this variable space is "poor." (b) The framework of the proposed FedACD method (Federated Adaptive Causal Discovery) is depicted. (c) Experimental results on benchmark Bayesian network datasets: A total of 5,000 samples are evenly distributed across {3, 5, 10, 15, 20} clients. The performance of all methods is evaluated on two metrics: Structural Hamming Distance (SHD) and F1 score (from top to bottom). (d) Experimental results on synthetic non-IID datasets: A total of 5,000 samples are evenly distributed across {3, 5, 10, 15, 20} clients. The performance of all methods is shown on the same two metrics: Structural Hamming Distance (SHD) and F1 score (from top to bottom).
Federated Causal Discovery aims to uncover causal relationships among variables from distributed data across multiple clients while preserving data privacy. In practice, the quality of local data samples within different variable spaces can vary across clients, a phenomenon referred to as sample quality heterogeneity. As a result, data from different clients may be more suitable for learning causal relationships among specific variables. Existing federated causal discovery methods rely on aggregating all model parameters from every client, making them incapable of addressing the issue of sample quality heterogeneity. To overcome this limitation, this paper proposes a Federated Adaptive Causal Discovery (FedACD) method. During federated model aggregation, FedACD adaptively selects causal relationships learned from "good" variable spaces (i.e., spaces with high-quality samples) on each client while masking those from "poor" variable spaces (i.e., spaces with low-quality samples). In this way, each client only needs to send its optimal learning results to the server, enabling accurate federated causal discovery. Extensive experiments on various datasets demonstrate that the proposed method significantly outperforms existing approaches.