In December 2024, Wang Zihan, a master's student from the Media Computing Laboratory at the School of Computer Science and Information Engineering, Hefei University of Technology (supervised by Professor Wu Le), and their collaborators had their research paper titled “Making Non-overlapping Matters: An Unsupervised Alignment-enhanced Cross-Domain Cold-Start Recommendation” accepted as a full-length article by the internationally renowned journal IEEE Transactions on Knowledge and Data Engineering (TKDE).
Paper Title: Making Non-overlapping Matters: An Unsupervised Alignment-enhanced Cross-Domain Cold-Start Recommendation
Authors: Zihan Wang, Yonghui Yang*, Le Wu*, Richang Hong, Meng Wang
Paper Link: https://ieeexplore.ieee.org/document/10779184
Cold-start recommendation is a long-standing challenge when presenting potential preferred items to new users. Most empirical studies leverage side information to promote cold-start recommendation. In this work, we focus on cross-domain cold-start recommendation, which aims to provide suggestions to those non-overlapping users who have only interacted in the source domain and are viewed as new users in the target domain. Pre-training and then mapping is the common solution for the cross-domain cold-start recommendation. The former learns domain-specific user preference, and the latter transfers preference knowledge from the source to the target domain. Despite the effectiveness, we argue that current mapping-based methods still have the following limitations. First, current mapping functions fail to fully consider the similarity of user behavioral patterns, either common transfer or personalized transfer mappings. Second, sparse supervision signals from the limited overlapping users, lead to insufficient mapping function learning for recommendation. To tackle the above limitations, we propose a novel MACDR model for cross-domain cold-start recommendation. Specifically, MACDR consists of two elaborate modules: a Prototype enhanced Mixture-Of-Experts (PMOE) based mapping function and a Preference Distribution Alignment (PDA) enhanced optimization. PMOE is designed to balance the transfer patterns of common and personalized preferences, following the basis that similar users share similar preference transfer. Furthermore, to alleviate the sparse supervision issue, PDA is designed to explore the utilization of non-overlapping users in an unsupervised manner based on the prototype distribution alignment technique. Extensive experiments on three real-world datasets demonstrate the effectiveness of the proposed method.
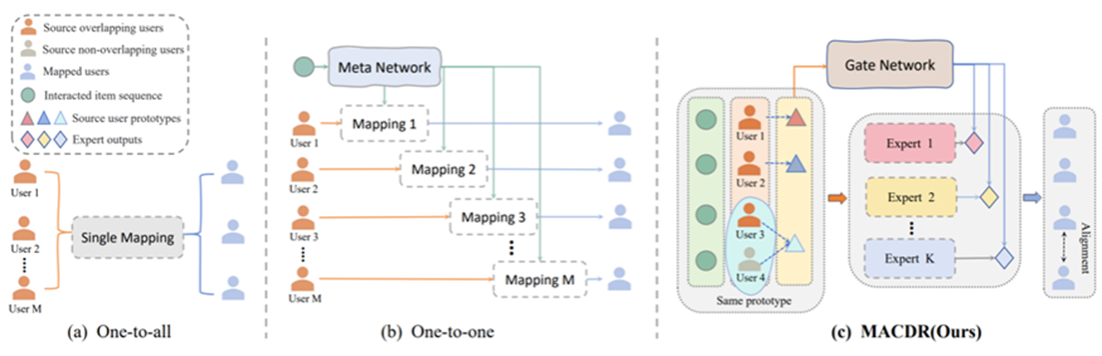
Fig. 1: Illustration of three mapping functions. (a) One-to-all [25, 18, 22]: all users share the same mapping function; (b) One-to-one [51, 41]: each user has a personalized mapping function; (c) MACDR (Ours): we propose a Prototype enhanced Mixture-Of-Experts (PMOE) based mapping function to capture the transfer patterns of common and personalized preferences and design an unsupervised preference distribution alignment (PDA) optimization to better exploit the nonoverlapping users, following the assumption that users with the same prototype have closer representation after mapping
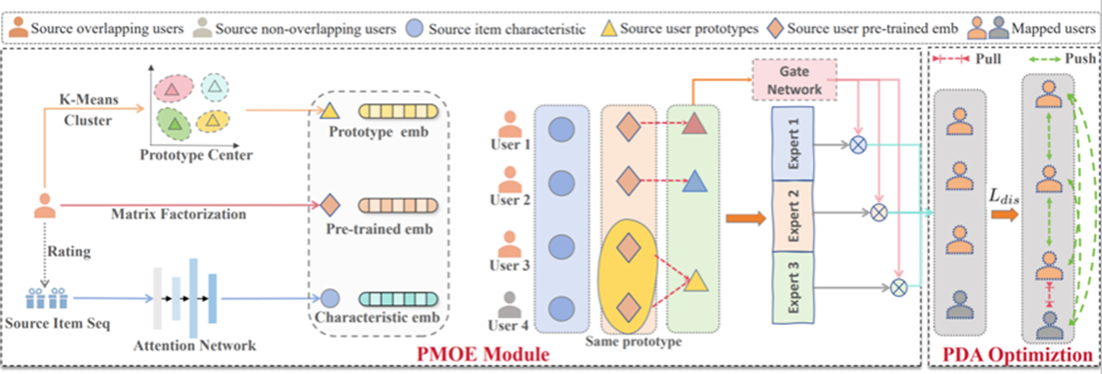
Fig. 2: An Illustration of our proposed MACDR framework, which consists of Prototype enhanced Mixture-Of Experts (PMOE) mapping module and Preference Distribution Alignment (PDA) optimization strategy. The PMOE module provides a flexible mapping function that combines the patterns of common and personalized preference transfer based onuser prototypes. The PDA optimization strategy utilizes non-overlapping users to boost recommendation performance in an unsupervised manner
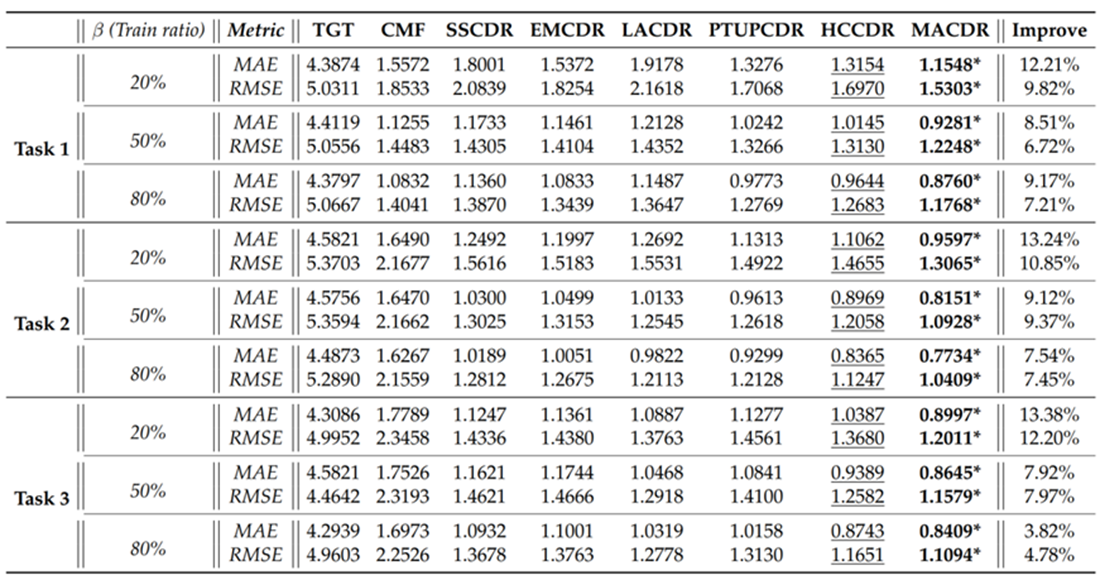
TABLE 1: Recommendation performance of our proposed MACDR and other baselines. For MAE and RMSE metrics, the lower value denotes better performances. The best results are in boldface and the second-best results are in underline. ∗indicates 0.05 level, paired t-test of MACDR (Ours) vs. the best baselines. Improve denotes relative improvement over the best baseline
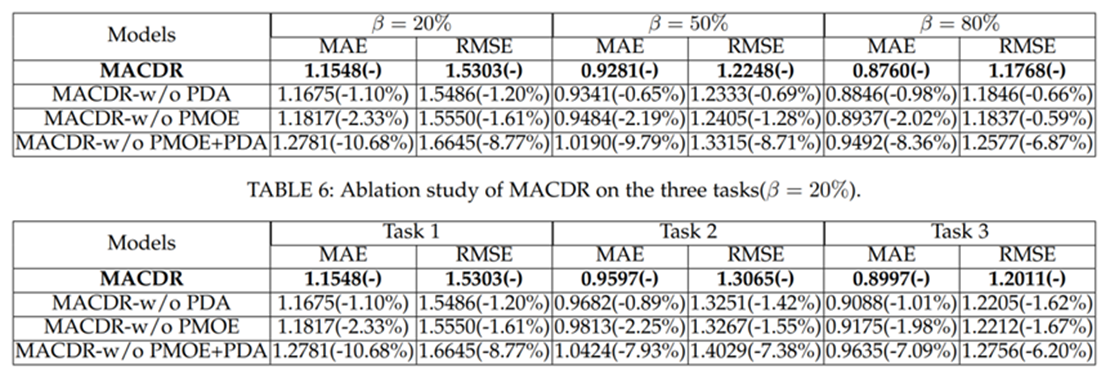
TABLE 2: Ablation study of MACDR on the Sports-Clothing dataset(task1). ”MACDR-w/o PDA” denotes removing the PDA optimization of MACDR. ”MACDR-w/o PMOE” denotes using a linear mapping function instead of PMOE for MACDR. ”MACDR-w/o PMOE+PDA” denotes removing PDA and PMOE modules
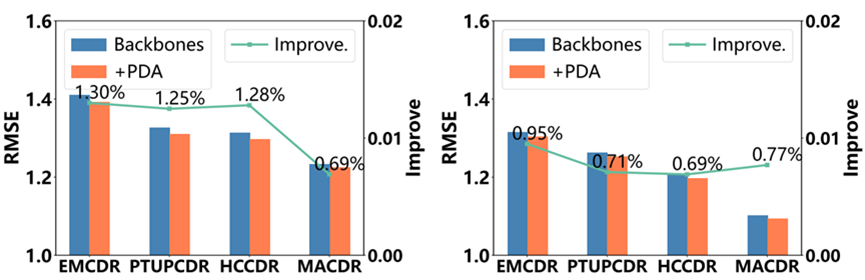
Fig. 3: Generality of PDA combined with various backbones
IEEE Transactions on Knowledge and Data Engineering (TKDE) is a prestigious international journal in the field of knowledge discovery and data mining. It focuses on publishing the latest research advances in areas such as databases, data mining, knowledge modeling, and distributed data management systems. TKDE is recognized as an A-level journal by the China Computer Federation (CCF), and its latest impact factor is 8.9.