Sign language, a silent miracle of communication, is not only a shining gem in academic research but also a golden key for the deaf community to integrate into society. However, the leap forward in sign language technology has been hindered by the stumbling block of data scarcity. The team led by TANG Shengeng at our school has ignited hope with creativity—inventing a method to weave discrete sign language gestures into fluent videos, paving a new path to address the challenge of insufficient sign language data.
Recently, the research achievement of TANG Shengeng from the School of Computer Science and Information Engineering at Hefei University of Technology, titled “Discrete to Continuous: Generating Smooth Transition Poses from Sign Language Observations,” was accepted and published at the IEEE Conference on Computer Vision and Pattern Recognition (CVPR), a top-tier international conference in computer vision. CVPR is widely recognized as the premier academic conference in the field of computer vision and is rated as a Category A conference by the China Computer Federation (CCF). As one of the most influential academic events in computer vision, CVPR enjoys an exceptional reputation worldwide, and the research it publishes typically represents the highest standards in the field.
Paper Title: Discrete to Continuous: Generating Smooth Transition Poses from Sign Language Observations
Authors: TANG Shengeng, HE Jiayi, CHENG Lechao, WU Jingjing, GUO Dan, HONG Richang
Paper Link: https://arxiv.org/abs/2411.16810
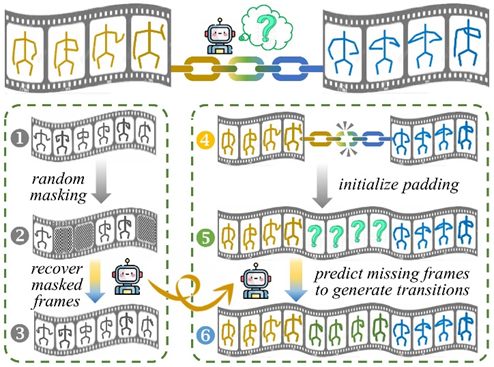
Figure 1. Task and key steps. Our work aims to generate continuous sign videos by creating transition poses between discrete segments. In training, random masking simulates missing transitions, and the model learns to recover these frames (steps 1-3). During inference, padding initializes missing transitions, which the model refines to generate smooth, coherent sequences (steps 4-6)
Abstract:Generating continuous sign language videos from discrete segments is challenging due to the need for smooth transitions that preserve natural flow and meaning. Traditional approaches that simply concatenate isolated signs often result in abrupt transitions, disrupting video coherence. To address this, we propose a novel framework, Sign-D2C, that employs a conditional diffusion model to synthesize contextually smooth transition frames, enabling the seamless construction of continuous sign language sequences. Our approach transforms the unsupervised problem of transition frame generation into a supervised training task by simulating the absence of transition frames through random masking of segments in long-duration sign videos. The model learns to predict these masked frames by denoising Gaussian noise, conditioned on the surrounding sign observations, allowing it to handle complex, unstructured transitions. During inference, we apply a linearly interpolating padding strategy that initializes missing frames through interpolation between boundary frames, providing a stable foundation for iterative refinement by the diffusion model. Extensive experiments on the PHOENIX14T, USTCCSL100, and USTC-SLR500 datasets demonstrate the effectiveness of our method in producing continuous, natural sign language videos.