The rapid advancement of multimodal large models has rendered the generation and dissemination of false information increasingly covert and complex. In response to this challenge, we have proposed a novel multimodal manipulation detection and grounding method—ASAP. Leveraging semantic enhancement technology, it precisely captures tampered content and integrates the Manipulation-Guided Cross Attention (MGCA) mechanism, enabling the model to perform faster and more accurately in the race of information discernment. Experimental results on the DGM4 dataset demonstrate that this method significantly outperforms existing technologies, establishing a robust defense line for information security. Come and witness how the "Guardian of Truth" in the AI era plays its role.
Recently, a research paper by a master's student from our school was accepted by the IEEE/CVF Conference on Computer Vision and Pattern Recognition (CVPR), a top international conference in computer vision. This study was guided by Associate Professor Wang Yaxiong, who also serves as the corresponding author. CVPR, being one of the most influential academic conferences in the global computer vision field, attracts tens of thousands of paper submissions each year. The acceptance of this paper also showcases our institute's research strength in the field of multimodal artificial intelligence.
Paper Title: ASAP: Advancing Semantic Alignment Promotes Multi-Modal Manipulation Detecting and Grounding
Authors: Zhenxing Zhang, Yaxiong Wang, Lechao Cheng, Zhun Zhong, Dan Guo, Meng Wang
Paper Link: https://arxiv.org/pdf/2412.12718
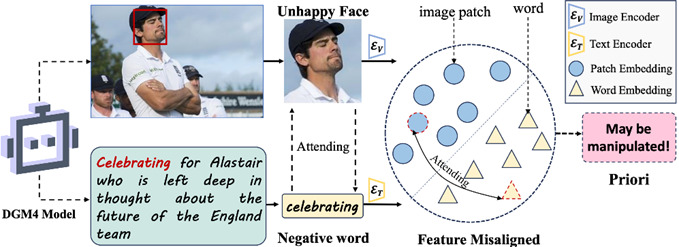
Figure 1. Fine-grained understanding of the multimodal media is one of keys for detecting the manipulated media. The capture of the misaligned components between the image and the text can effectively assist the DGM4 task
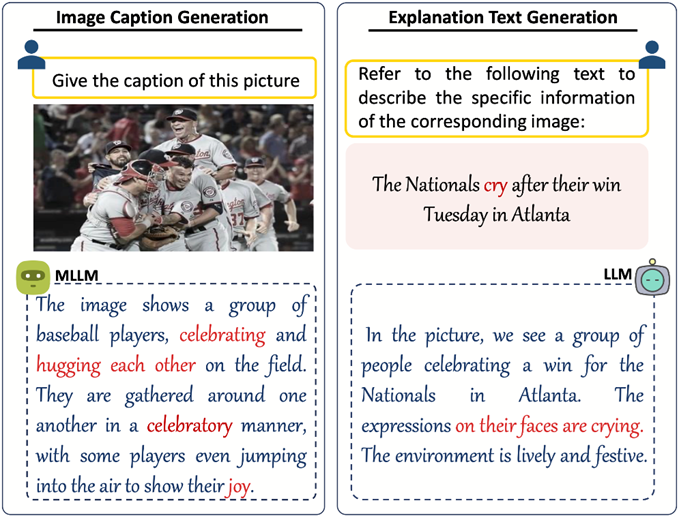
Figure 2. Illustration of the generation of image caption (left) and explanation text (right). The auxiliary texts can be effectively harvested via the off-the-shelf large models with the carefully crafted instructions
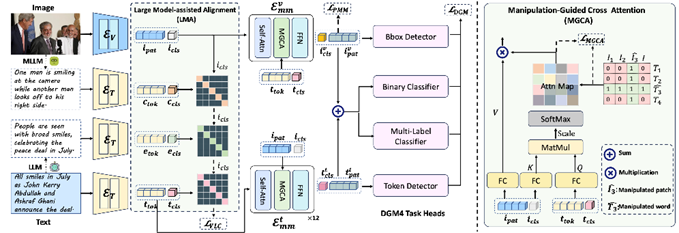
Figure 3. Illustration of our proposed ASAP framework. We employ a Multimodal Large Language Model (MLLM) to generate captions and a Large Language Model (LLM) to produce explanation texts for social media image-text pairs. These, along with the image, are encoded to obtain feature representations. Our Large Model-assisted Alignment (LMA) module enhances cross-modal alignment, followed by two Multimodal Encoders with Manipulation-Guided Cross Attention (MGCA) to integrate features for task-specific representations. One encoder is vision-biased for image grounding, and the other is text-biased for text grounding. The combined features from both encoders are used for media authenticity detection and manipulation identification. The network is optimized using DGM losses and objectives from LMA and MGCA.
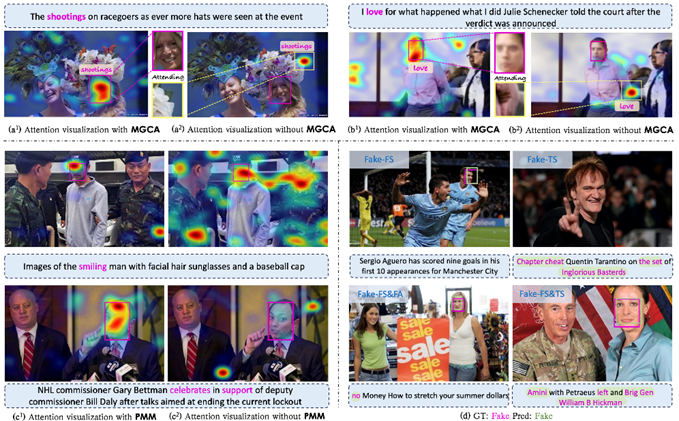
Figure 4. Effect of MGCA and PMM Loss on Attention Map Visualization. The red rectangle represents the bounding box of the manipulated face, and the red text indicates the manipulated word. (a) and (b) show the attention visualization between the manipulated word and the image. (c) shows the attention visualization between the entire sentence and the image. (d) presents the model’s prediction compared to the Ground Truth.
Abstract:We present ASAP, a new framework for detecting and grounding multi-modal media manipulation (DGM4). Upon thorough examination, we observe that accurate finegrained cross-modal semantic alignment between the image and text is vital for accurately manipulation detection and grounding. While existing DGM4 methods pay rare attention to the cross-modal alignment, hampering the accuracy of manipulation detecting to step further. To remedy this issue, this work targets to advance the semantic alignment learning to promote this task. Particularly, we utilize the off-the-shelf large models to construct paired image-text pairs, especially for the manipulated instances. Subsequently, a cross-modal alignment learning is performed to enhance the semantic alignment. Besides the explicit auxiliary clues, we further design a ManipulationGuided Cross Attention (MGCA) to provide implicit guidance for augmenting the manipulation perceiving. With the grounding truth available during training, MGCA encourages the model to concentrate more on manipulated components while downplaying normal ones, enhancing the model’s ability to capture manipulations. Extensive experiments are conducted on the DGM4 dataset, the results demonstrate that our model can surpass the comparison method with a clear margin. Code will be released at https://github.com/CriliasMiller/ASAP.