Knowledge graph logical querying aims to leverage interpretable knowledge to provide reliable reasoning results for complex questions, which is crucial in high-stakes decision-making scenarios such as medical diagnosis and financial risk assessment. However, existing logical reasoning algorithms for knowledge graphs often struggle to capture the underlying logical semantics between conditions in complex queries, severely limiting reasoning performance. To address this challenge, our study proposes QIPP (Query Instruction Parsing Plugin), a plug-and-play module that enhances various knowledge graph reasoning algorithms by enriching their logical semantic understanding.
Recently, the paper "Effective Instruction Parsing Plugin for Complex Logical Query Answering on Knowledge Graphs"—authored by Xingrui Zhuo (Ph.D. candidate, supervised by Prof. Xindong Wu) from our school, in collaboration with researchers from Beijing University of Technology and Griffith University, Australia—has been accepted by The ACM Web Conference (WWW).
Paper Title:Effective Instruction Parsing Plugin for Complex Logical Query Answering on Knowledge Graphs
Authors:Xingrui Zhuo, Jiapu Wang, Gongqing Wu, Shirui Pan, Xindong Wu
Paper Link:https://doi.org/10.1145/3696410.3714794
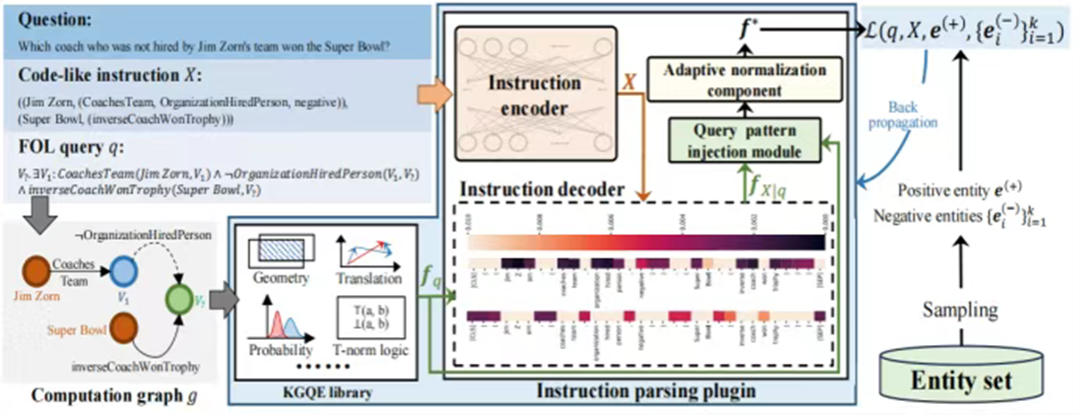
Figure 1. Framework diagram of the QIPP model
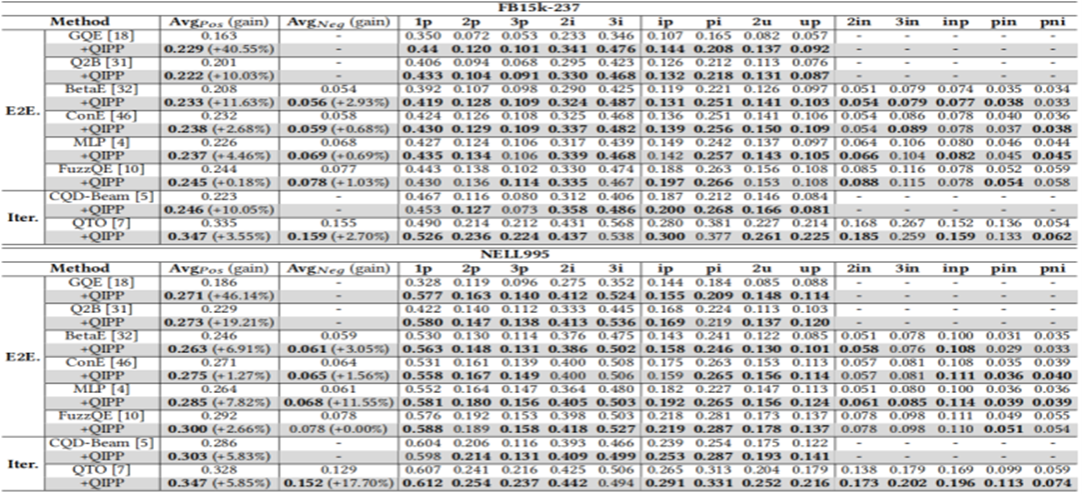
Table 1: MRR of KGQE models with QIPP. "E2E." and "Iter." represent end-to-end and iterative KGQE models, respectively. The bold font indicates that a KGQE model has higher MRR values after adding QIPP
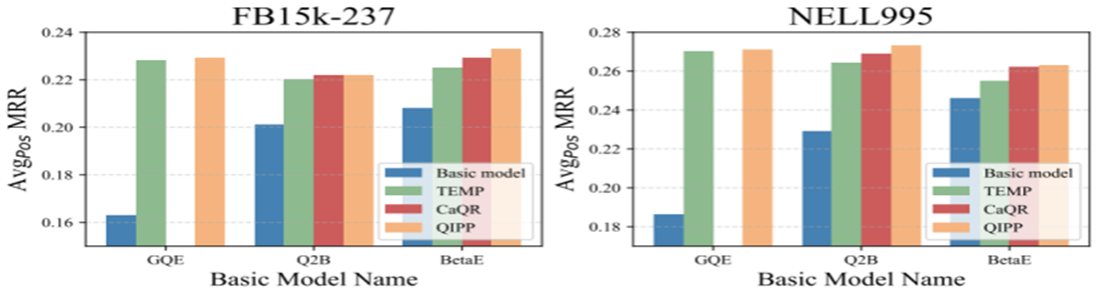
Figure 2: Comparison between QIPP and different QPL plugins. Since GQE and FB15k are not used in CaQR’s experiments, we only present the basic models and datasets shown in the above figure
Abstract: Knowledge Graph Query Embedding (KGQE) aims to embed FirstOrder Logic (FOL) queries in a low-dimensional KG space for complex reasoning over incomplete KGs. To enhance the generalization of KGQE models, recent studies integrate various external information (such as entity types and relation context) to better capture the logical semantics of FOL queries. The whole process is commonly referred to as Query Pattern Learning (QPL). However, current QPL methods typically suffer from the pattern-entity alignment bias problem, leading to the learned defective query patterns limiting KGQE models’ performance. To address this problem, we propose an effective Query Instruction Parsing Plugin (QIPP) that leverages the context awareness of Pre-trained Language Models (PLMs) to capture latent query patterns from code-like query instructions. Unlike the external information introduced by previous QPL methods, we first propose code-like instructions to express FOL queries in an alternative format. This format utilizes textual variables and nested tuples to convey the logical semantics within FOL queries, serving as raw materials for a PLM-based instruction encoder to obtain complete query patterns. Building on this, we design a query-guided instruction decoder to adapt query patterns to KGQE models. To further enhance QIPP’s effectiveness across various KGQE models, we propose a query pattern injection mechanism based on compressed optimization boundaries and an adaptive normalization component, allowing KGQE models to utilize query patterns more efficiently. Extensive experiments demonstrate that our plugand-play method1 improves the performance of eight basic KGQE models and outperforms two state-of-the-art QPL methods.
The ACM Web Conference (WWW) is one of the world’s premier annual academic conferences, dedicated to advancing research on the World Wide Web and its related technologies. Organized by the International World Wide Web Conference Committee (IW3C2) in collaboration with ACM SIGWEB, the conference rotates annually among North America, Europe, and Asia. Recognized as a CCF-A conference (the highest rank in the China Computer Federation's classification), WWW holds exceptional prestige in academia. With an acceptance rate of just 19.8% in 2025, the conference maintains rigorous review standards and upholds a reputation for cutting-edge scholarly quality.